How The Wall Street Journal Prioritizes Tests for Higher Experimentation ROI
Experimenting is an essential tool for increasing revenue, but how do you know what tests to run? Prioritizing tests is key for getting higher and quicker return once you have decided to make the investment of experimenting. But, learning which tests to prioritize can seem daunting to new experimenters. With hundreds of potential tests and
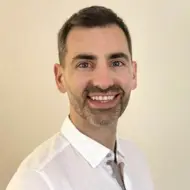
Steven Schuler
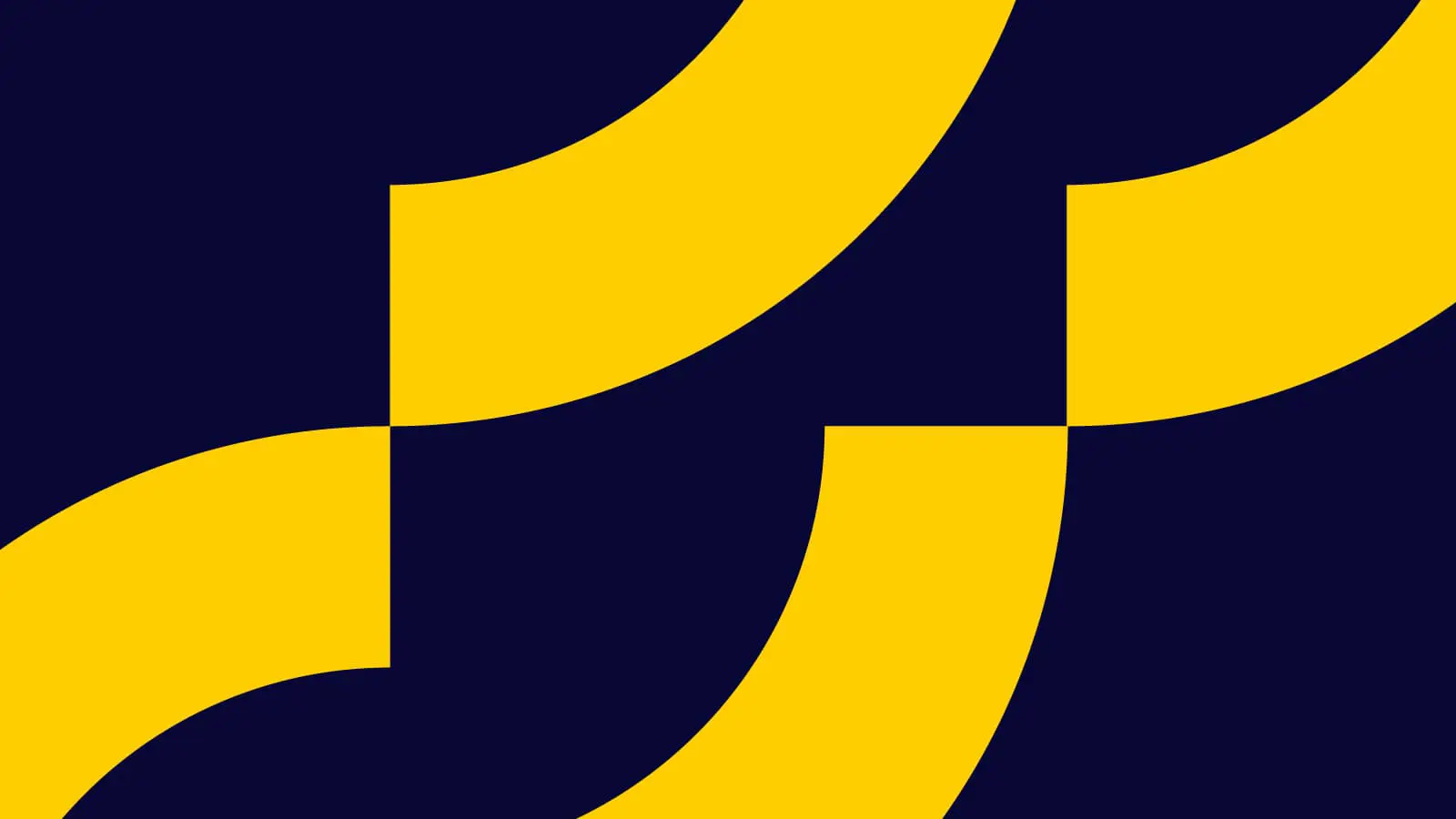
Experimenting is an essential tool for increasing revenue, but how do you know what tests to run? Prioritizing tests is key for getting higher and quicker return once you have decided to make the investment of experimenting. But, learning which tests to prioritize can seem daunting to new experimenters. With hundreds of potential tests and little data at the start, how can teams discover which tests are most worth running?
In this article, we’ll share tips from Wall Street Journal’s Olivia Simon, Optimization Manager. Her team is responsible for experimentation on The Wall Street Journal and Barron’s. Together with stakeholders across the business, the optimization team experiments across acquisition, engagement, product and membership retention – leading the process from test ideation to launch to impact after completion.
Olivia shared her knowledge with us in a recent webinar, Optimizing Membership at The Wall Street Journal. We’ll cover the steps to prioritizing tests as well as how prioritization improved WSJ’s experimentation ROI.
Step 1. Find a test with more than a 3% expected uplift.
The first step WSJ takes is to find a test where the team expects more than 3% uplift stating, “If we think something might have less than 3% impact, we rule it out.” How do you know what will have more than a 3% impact? Olivia says — “We reference previous tests and onsite data, to make an educated guess. The data we have helps us to make an informed projection of what might happen .”
To guess which tests might have the highest impact, the team first comes up with many potential experiments. At WSJ, test ideas can come from, “anyone and everyone.” Having more teammates and departments involved in the process not only ensures the adoption of an experimentation culture. It also makes tests more diverse.
Step 2. Multiply the expected uplift by the traffic, monetary value, and conversion rate.
Once they’ve got an idea of an experiment’s potential uplift, the team multiplies that uplift by the traffic flowing through the experience. For example, if 100,000 users click through to your shopping cart every day, then you’d multiply your expected uplift by 100,000.
Next, WSJ multiplies that traffic by the monetary value of the KPI. So, if you’re optimizing clicks to your cart, you need to determine what those clicks are worth by assigning a dollar amount to them. Let’s walk through assigning a dollar amount to a KPI.
First, analyze the data you have:
- What is your average checkout value? Let’s say it’s $100.
- How many clicks are in your checkout process? For our example, let’s go with 4 clicks.
- How much more valuable is the last click vs. the other clicks? Let’s say that we consider the 3rd click to be 2X more valuable than the first 3 clicks since it’s the last step in the checkout process. Or, you may want to keep each click equal in value.
Next, to determine our click (KPI) dollar value, we use a simple formula like this:
- If we assigned equal value to each click, then a click is worth $25. (100/4)
- If we assign 2X value to the last click, then the last click is worth $40, and the previous 3 are worth $20.
Example
So, let’s say we expect a 5% uplift in traffic for a new checkout process that currently gets 100,000 clicks. And, we want to assign equal value to each click in our checkout process (4 clicks) for our average purchase price of $100.
(100,000 x 5%) x $25 = $125,000
Step 3. Subtract the level of effort as a dollar amount.
Now that we know we expect $125,000 in increased checkout from our experiment, it’s time to deduct the cost of running the experiment. To do this, we subtract the dollar amount of “effort” or human time put into the experiment. Let’s look at an example of calculating effort.
Let’s say you have a team of 4 responsible for running the experiment: 2 engineers making $100,000 per year and 2 experimenters making $100,000 per year. If we assume each works 40 hours per week, then we’re looking at an hourly cost of about $48.
For this example, we’ll assume the experiment will take 3 hours of each teammate’s time.
3 hours x 4 people x $48 = $576 total effort
Step 4. Evaluate the return.
The final step is to subtract the total effort from the total expected revenue from the uplift.
$125,000 – $576 = $124,424 total ROI for this test
Based on our example, we’d run a test that would take our team a total of 12 human hours to implement. And, we’d make an estimated $124,424. So, this test would be absolutely worth our time. Unless of course, there was another test we could run with higher returns. But this would effectively be a quick win and worth running.
WSJ prioritized early tests for quick wins.
One of WSJ’s early wins was a visual test. They decided to test adding “you can cancel anytime” to their checkout page. This initial test increased subscriptions by 10%.
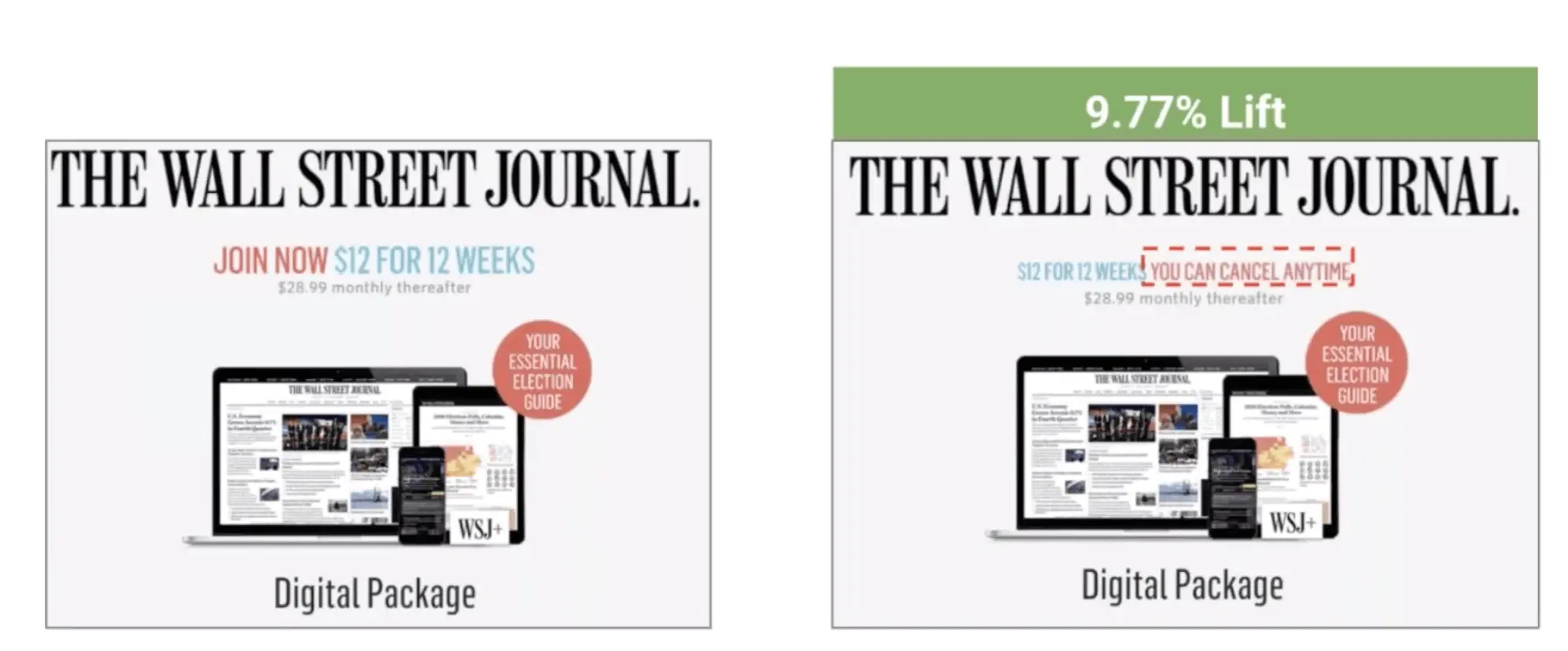
VARIANT (ON THE RIGHT) ADDED SIMPLE CANCELLATION MESSAGING
Another test decreased the checkout steps from 20 to 15. Eliminating 5 steps decreased average checkout time by 15 seconds and improved conversions by 13%.
Key takeaways
Make sure you focus on the tests that will bring the highest returns, especially when you’re getting started with experimentation. Consider WSJ’s advice of not wasting time on tests that won’t have more than 3% uplift. And finally, plug your data into the process we’ve shared to determine the returns of each prospective test to know which are best to execute.