Lessons We Learned from Email A/B Tests in 2014
This year, the Marketing Automation team at Optimizely got serious about tracking our email A/B tests. In 2015, Optimizely will be taking experience optimization to the next level, and continue to rigorously test campaigns in order to provide the best experience for our customers. Looking back at some A/B tests we ran this year, here are some lessons learned, along with where I hope to take our email experiments in 2015.
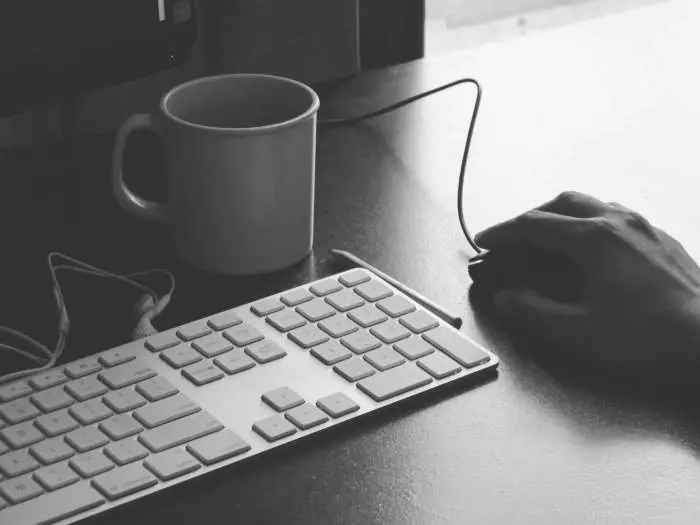
Bonjour tout le monde. This year, the Marketing Automation team at Optimizely got serious about tracking our email A/B tests. In 2015, Optimizely will be taking experience optimization to the next level, and continue to rigorously test campaigns in order to provide the best experience for our customers.
Looking back at some A/B tests we ran this year, here are some lessons learned, along with where I hope to take our email experiments in 2015.
Lesson 1: Creating a solid hypothesis is vital.
A successful email experiment not only allows you to make a decision at that point in time, but it also provides you with a new piece of knowledge that you can apply in future email sends. If the winning variation only applies to the test you just completed, you are missing out on an opportunity to get the most value out from your experiments.
I realized this while pulling the results of a particular test where the variation (FAQ: How long should my test run?) saw a 33.9% increase in clicks.
Subject line test
Control: Are you measuring your tests accurately? Measuring statistical significance of your test
Variation: FAQ: How long should my test run?
Stoked on this result, I went to post the findings in Optiverse, only to realize that I didn’t actually know what had made the variation successful. Did people click on this variation because they liked to read FAQs? Or because it was written in first person? Or something else entirely?
I looked to see what our hypothesis was upon creating the experiment, only to discover that we hadn’t written one.
:/
The first step to creating a solid hypothesis is to actually write it down before you run the test.
*If you are looking for a great resource on constructing a solid hypothesis, check out this blog post by Shana Rusonis. I’ve recommended this post probably 20 times already because it outlines what a foolproof hypothesis looks like, and provides recommendations on how to draw the most insights from your experiments.
So while this particular subject line test was inconclusive, we can always test again to generate clear results.
Let’s dig into this test a little deeper: our goal for this email was to to anticipate questions we often hear from new customers, and provide them with accessible resources. A solid hypothesis for this test could have been: If we pose a question in our subject line that mirrors how our customers think about a testing challenge, then clicks to our resource page will increase.
When thinking about the challenge from an experience optimization perspective, some followup questions arise: how do our customers find answers to questions? Is email the right way to deliver answers to common questions? What kind of customers engage with our resources through email?
Some follow-up test ideas for 2015:
- Target the audience:
- Hypothesis: The click through rate will be higher on FAQ emails for customers who have previously submitted support cases.
- Test resource placement:
- Hypothesis: Adding a FAQ article list in our help center next to the support submission form will decrease support tickets that are related to the FAQ.
These tests will help us understand what is important to our customers, and how to best communicate with them.
Lesson 2: Statistically insignificant experiments can drive business decisions.
Working at a startup, we are constantly vigilant of the balance between adopting new online marketing trends and keeping our process lean and dynamic. We are often inspired to try out new products, integrations, and tools to deliver better experiences to our customers. However, every new integration requires training and technical setup, and often means more review time for each email sent. AB tests are super helpful to gauge whether the new integration/product/ feature is worth the investment.
#BBD0E0 »