Customer experience analytics: metrics, data sources & solutions
Do you want to leverage the power of data-driven decision-making through customer experience analytics? Learn what you need to know in this guide.
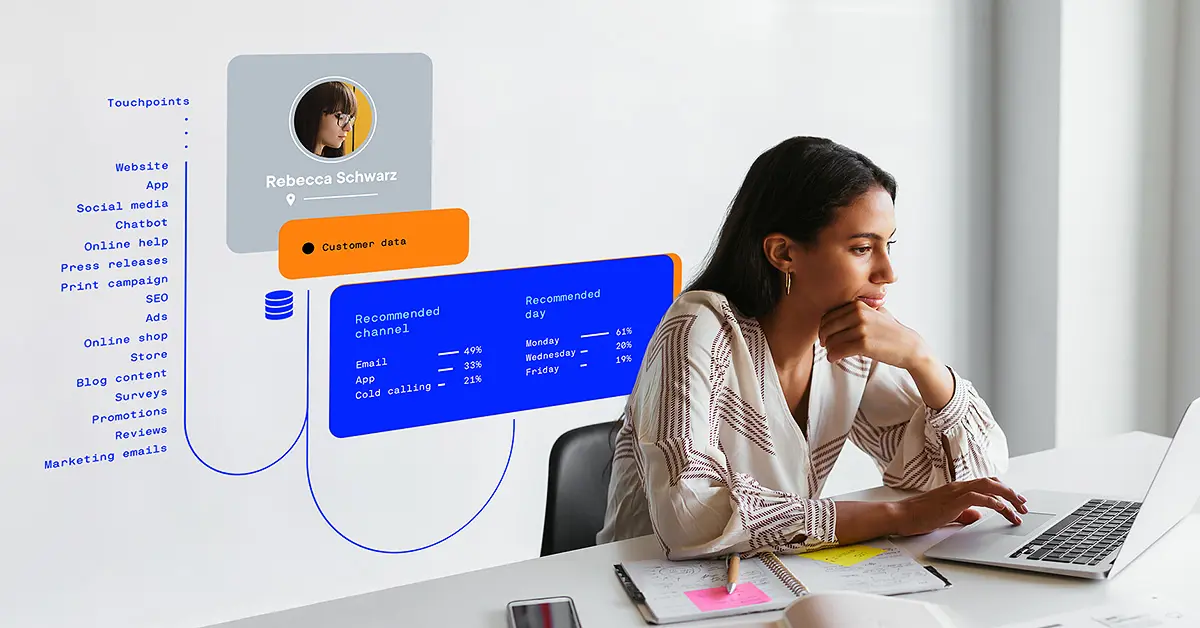
In the era of digital transformation and omnichannel communication, exceptional customer experience has become vital to a brand’s immediate and long-term success. In 2021, 49% of consumers reported changing brands over subpar customer experiences. As options proliferate and changing brands—in both B2C and B2B contexts—continues to be easier than ever before, data-driven decision-making in customer experience management remains a primary challenge for brands keen to thrive and stand out in the crowd.
Decision-makers have not forgotten about the escalated import of customer experiences. 86% of customer experience design professionals agree that customer experiences define the nature of their interaction with the competition. 87% of business leaders identify customer experience as their top potential growth engine, but only a third feel prepared to field this challenge.
The churn of bad—yet avoidable—customer experiences costs US businesses $35.3 billion annually. Businesses need meaningful customer experience analytics to get a handle on these losses and seize the opportunities they create. In this guide, you’ll learn what data-driven decision-making means in the digitally transformed world and how to establish the metrics, data sources, and solutions you need.
Key takeaways
- The data on customer loyalty and habits indicates that providing exceptional customer experiences is more important now than ever before.
- In the digital era, businesses can use tools to turn their customer and transactional data into predictive and actionable insights that can improve decision-making processes.
- Business leaders can learn how to gain and apply these insights through examples of successful data-driven decision-making guided by customer experience analytics
What is data-driven decision-making?
Before the digitization of business data, decision-makers in most businesses had no real tools for effective data management. Operations ran manually, documentation passed into folders and filing cabinets, and—at best—clerical staff put as much as they could into alphabetical order. Businesses stored this data for the sake of record-keeping and liability mitigation. The process did not generate insight or provide decision-makers with meaningful metrics to make informed choices and enhance operations at the bottom line.
Everything changed when the digital transformation took this data out of filing cabinets and made it globally visible. Businesses developed automated tools to interpret and process their data. With modern analytical tools, decision-makers can integrate real-time comprehensive customer data into their processes and make choices guided by observable trends in information.
Business processes thrive with goal orientation. This is nowhere more evident than in data collection. Customer and transaction data can exist passively in an organization simply as records – and perhaps as mitigation of legal and audit risks – or you can apply the right tools to extract, interpret and visualize what it represents to create a more informed conception of your processes and client relationships.
Customer experience analytics
Customer-focused data-driven decision-making in practice yields customer experience analytics. Customer experience analytics refers to gathering and analyzing data from customers to better understand their needs, perspectives and experiences with your products and services. Analytics can help you increase customer engagement and loyalty, better understand and improve your customer experience.
Examples of customer experience analytics
Customer experience analytics encompasses the full range of the customer journey within your organization. The data sources of these analytics can be direct or indirect.
Direct customer experience analytics include:
- Net promoter scores
- Customer satisfaction ratings
- Customer effort scores
- Open text comments in surveys and product reviews
- Social media interactions
Indirect customer experience analytics include:
- Customer lifetime value
- Average order value
- Customer renewal rates
- Churn rates
- Average handle times
Data-driven customer experience analytics in action
To better understand what data-driven decisions derived from customer experience analytics look like in the real world, it helps to take a closer look at some notable examples. Here is a list of recent meaningful applications of analytics that yielded demonstrable value.
1. Uber’s AI-driven car distribution and pricing
For transportation service providers, hiring drivers, establishing a fleet and connecting them with clients no longer suffices to create competitive customer experiences. Transportation service providers also have to reckon with the demand-supply gap that emerges when there is a high variance of popularity in different city locations.
To mitigate this potential service gap, Uber uses real-time AI-driven data streams of fleet locations and service demand trends. Uber’s AI determines the optimal geographic distribution of drivers in the fleet and adjusts pricing automatically to match peak hours. This allows the company to meet customer expectations seamlessly as they change in real-time.
2. Amazon product recommendations
Data-driven technology allows for a more tailored customer experience. E-commerce platforms rely on this functionality to keep their customers engaged. Amazon deploys data-driven AIs to craft highly personalized product recommendations. The AI examines what customers have put in their shopping carts and what they have previously purchased from Amazon. It also collects information on client reviews and the pages they visit. The shop can generate recommendations with significantly higher purchase rates by combining these data points.
3. Google’s people analytics
Unsurprisingly, Google relies heavily on data science throughout its algorithm design processes. However, Google also applies data-driven decision-making processes in human resources. The company maintains a department for People Analytics. The department exists to collect data for measuring the effects of managerial staff on people. The idea is to use internal performance data correlated with management activities to better understand the key indicators of good management and to adjust manager evaluations to improve productivity.
4. Starbucks’ digital flywheel program
Starbucks’ rewards program and mobile app gather broad information about customers’ buying habits, preferences, and even behaviors based on the time of day and the weather. When customers visit new locations, this information is available to team members through the customer’s smartphone ID to provide highly personalized recommendations and deliver the same quality customer experiences regardless of the location. Starbucks’ Digital Flywheel Program—a cloud-based AI—drives the collection and presentation of this data in real-time and enables teams in all locations to treat customers they may have never interacted with before as familiar, valued individuals.
AI-driven content management with Optimizely
Ready to use data-driven analytics and AI to take your customer digital experiences to the next level? Optimizely has established trusting relationships with over 9,000 leading companies for digital experiences, content management, experimentation and commerce.
To view a demo of our content management system, contact us today.