5 ways AI helps you get market research right
Today’s market research depends on data-driven decision-making frameworks and AI for improved organizational performance. Here’s how AI can help you.
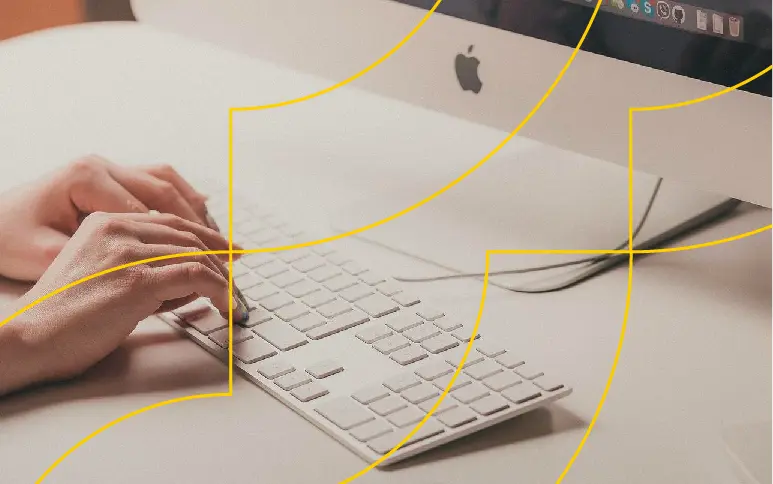
Organizations that use a data-driven approach when making business decisions can outperform competitors and leverage new strategies for improved results. Most businesses depend on a sound business strategy to survive in challenging business climates, and adopting a data-driven decision-making process can make all the difference.
The advent of artificial intelligence (AI) to analyze large sets of data and make intelligent inferences about your business is becoming common practice in boardrooms around the world. AI is helping thousands of organizations to optimize functions like marketing, sales and customer service.
Today, we’ll look at five ways that you can get your market research right using AI and data-driven decision-making in your business.
Key takeaways:
- AI can help contextualize your data and allow you make better business decisions quicker
- To get the most out of your data-driven decision-making framework, you should start with a clear business strategy
- You can use AI and analytics engines to uncover new insights and build a strategy that can outperform your competitors
- While AI can assist with your business decision-making process, remember that it won’t replace human judgment entirely
5 ways to use AI for data-driven decision making
Advances in AI technologies are helping modern organizations to discover new insights into all elements of their business. By using AI to do much of the heavy lifting, your business can make intelligent business decisions quicker than ever before.
Every transaction, customer interaction, social engagement or microeconomic indicator you can think of is available to use in your analytical framework. While this increases the reaction speed with which you can make decisions, it also removes any cognitive biases from the process.
Modern business intelligence (BI) frameworks now regularly deploy AI and data-driven algorithms as part of the analytics process to classify, segment and contextualize data into actionable information. Below, we’ll go over five tips that you can use to get the most out of your AI decision-making framework and make data-driven business decisions.
1. Start with a formalized data-driven decision making strategy
Firstly, you need to formalize a strategy for when and how you’ll need to use AI in your business decision-making processes. Consider the decision-making model indicated below.
The framework above uses machines to analyze large sets of data, but the output is still going through a filter that depends on human judgment. A better way to make data-driven business decisions is to use machines for the entire process, and only use human intervention where necessary.
AI that uses a data-driven decision workflow can process non-linear relationships that transcend a human’s cognitive biases. To set your strategy and determine which workflow will work best, focus on your specific objectives and establish clear goals.
Start developing your strategy by answering questions like:
- What do we want to achieve with this framework?
- Where is the biggest growth potential for our brand?
- Are we setting our prices at the right level compared to other businesses?
- When is the best time to engage our customers about a specific product or service?
If you set out your specific goals with enough detail, you can avoid being overwhelmed by the sheer amount of data that you gather during your normal business processes.
2. Map your datasets to your business goals
A formalized strategy sets out the goals you want to achieve with your data-driven decision-making framework. After you’ve set your business goals, you can map your available datasets and identify any gaps in your analytics process.
You may have mounds of sales data but not enough insight into how your marketing efforts are resonating with prospective customers every day. Identify the data you have, what it means in a strategic context, and what else you need to make better business decisions in the future.
For the best results, you’ll need to frame your business goals with enough detail so it enables you to answer specific questions using a variety of data sources and analysis models. You can then experiment with different strategies to find an optimized framework that suits your business.
3. Collect only data that informs your business decisions
More data doesn’t necessarily mean smarter business decisions. If you have gaps in your current datasets, only gather additional data that will add value to your business goals. Organizations often make the mistake of thinking that bringing in more data will naturally start providing greater insight. By setting out your business goals and connecting only the data that helps you answer your strategic questions, you can reduce the workload.
You can assign your framework’s goals into three main categories:
- Intelligent insights – These questions are what help the C-suite make strategic decisions for the benefit of the organization. Intelligent advisors based on real-time data can assist the boardroom to invest in the right ideas and tools early.
- Operational excellence – For technical teams, the goal is to improve incrementally during each business cycle. Frame questions to uncover where you aren’t meeting your operational goals to establish your baselines of performance, and understand when to make strategic interventions.
- Hidden potential – Finally, AI’s capabilities continue to evolve and machine learning (ML) algorithms become smarter over time. You can use these features and capabilities to understand macroeconomic trends and position your brand for future success by uncovering hidden potential in your organization.
4. Determine the value of your AI and data-driven decision making framework
Review your strategy, datasets and insights regularly to understand what value the framework brings to your daily decision-making processes. The ideal outcome is to move from a descriptive-analytical model to a prescriptive AI-decision making framework.
By regularly revisiting your strategic business goals, you can establish which of your efforts yield real results, and where you can trim your approach due to little or no value. Prescriptive analytics can generate multiple courses of action for business decisions and rank these according to their statistical probability of succeeding. You can also use the outcome of these models as input for future data-driven decision-making.
5. Don’t discount the value of human intuition
The aim of AI-driven decision-making frameworks isn’t to automate the entire business process. To get the most out of your models and analysis, you need to combine the intelligence you gain from AI and data with the expertise of your human resources.
The diagram below demonstrates what a human and AI data-driven decision-making model may look like.
Constantly reconciling business decisions with the framework and extracting the best insights from both human resources and AI gives your business the best chance at success. By remaining curious and constantly experimenting with different strategies, you can drive engagement that outperforms your competitors.
Making data-driven decisions with AI and Optimizely
Optimizely is an experience platform that allows you to experiment, optimize and engage with your customers using data-driven strategies and business decisions. You can integrate your analytics engines and AI models with Optimizely to uncover new insights and track the effectiveness of your strategies in a single experience platform.
To see how Optimizely can assist you to make data-driven business decisions, get in touch with one of our offices today.