Stefan Thomke on Experimentation Culture and What Makes a Good Experiment
Previously we interviewed Harvard Business School Professor Stefan Thomke who recently published his book Experimentation Works: The Surprising Power of Business Experiments. Here in a second post related to his new book are powerful answers to why Experimentation Culture is so important and also a six-part outline of ‘What is a Good Experiment’. Additionally he
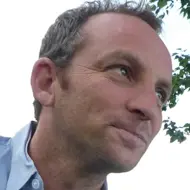
Todd Krieger
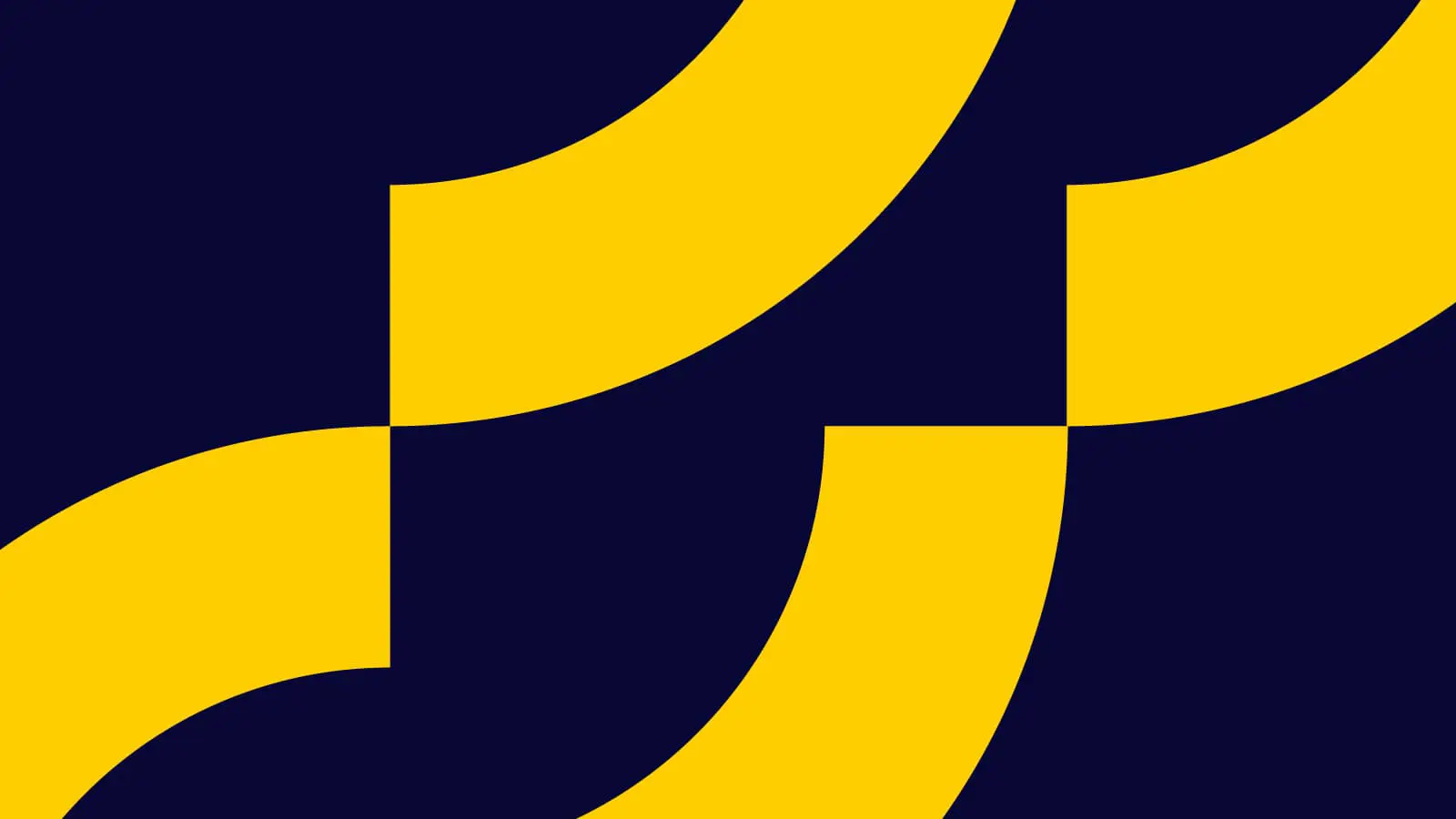
Previously we interviewed Harvard Business School Professor Stefan Thomke who recently published his book Experimentation Works: The Surprising Power of Business Experiments. Here in a second post related to his new book are powerful answers to why Experimentation Culture is so important and also a six-part outline of ‘What is a Good Experiment’. Additionally he was recently featured in the Harvard Business Review Magazine as well as on a podcast on these very topics.
Optimizely: Why is an Experimentation Culture so important and what are its most important attributes?
Stefan Thomke: Mark Okerstrom, the former CEO of Expedia Group once told me. “In an increasingly digital world, if you don’t do large-scale experimentation, in the long term—and in many industries the short term—you’re dead.” If testing is so valuable, why don’t companies do it more? After examining this question for several years, I can tell you that the central reason is culture. As companies try to scale up their online experimentation capacity, they often find that the obstacles are not tools and technology but shared behaviors, beliefs, and values. For every experiment that succeeds, nearly 10 don’t—and in the eyes of many organizations that emphasize efficiency, predictability, and “winning,” those failures are wasteful. To successfully innovate, companies need to make experimentation an integral part of everyday life—even when budgets are tight. That means creating an environment in which employees’ curiosity is nurtured, data trumps opinion, anyone (not just people in R&D) can conduct or commission a test, all experiments are done ethically, and managers embrace a new model of leadership. More specifically, this means:
Cultivate curiosity: Everyone in the organization, from the leadership on down, needs to value surprises, despite the difficulty of assigning a dollar figure to them and the impossibility of predicting when and how often they’ll occur. When firms adopt this mindset, curiosity will prevail and people will see failures not as costly mistakes but as opportunities for learning.
Insist That Data Trump Opinions: The empirical results of online experiments must prevail when they clash with strong opinions, no matter whose opinions they are. But it’s rare among most firms for an understandable reason: human nature. We tend to happily accept “good” results that confirm our biases but challenge and thoroughly investigate “bad” results that go against our assumptions.
Democratize Experimentation: To achieve scale, anyone at the company should be able to test anything—without management’s permission. This requires extensive training, transparency and ongoing discussions among team members about what the right problems are. Debates should be encouraged, and people should reach out to colleagues if they see anything that strikes them as questionable. Just as anyone can launch an experiment, anybody should be able to stop one.
Be Ethically Sensitive: When contemplating new experiments, companies must think carefully about whether users would consider the tests to be unethical. While the answer isn’t always clear cut, organizations that fail to examine this question risk sparking a backlash.
Embrace a Different Leadership Model: By democratizing experimentation and following test results where they lead, companies can enable employees to make good decisions on their own and accelerate innovation and improvements. But if most decisions are made this way, what’s left for senior leaders to do, beyond developing the company’s strategic direction and tackling big decisions such as which acquisitions to make? There are at least three things: 1) set a grand challenge that can be broken into testable hypotheses and key performance metrics, 2) put in place systems, resources, and organizational designs that allow for large-scale experimentation, and 3) be a role model, which means living by the same rule as everyone else and subjecting their own ideas to tests
Optimizely: What is a good experiment?
Stefan Thomke : In an ideal experiment, testers separate an independent variable (the presumed cause) from a dependent variable (the observed effect) while holding all other potential causes constant. They then manipulate the former to study changes in the latter. The manipulation, followed by careful observation and analysis, yields insight into the relationships between cause and effect, which ideally can be applied and tested in other settings. To obtain that kind of learning—and ensure that each experiment contains the right elements and yields better decisions—companies should ask themselves seven important questions: (1) Does the experiment have a testable hypothesis? (2) Have stakeholders made a commitment to abide by the results? (3) Is the experiment doable? (4) How can we ensure reliable results? (5) Do we understand cause and effect? (6) Have we gotten the most value out of the experiment? And finally, (7) Are experiments really driving our decisions? Although some of the questions seem obvious, many companies conduct tests without fully addressing them.
Here is a complete list of elements that you may find useful:
- Hypothesis
- Is the hypothesis rooted in observations, insights, or data?
- Does the experiment focus on a testable management action under consideration?
- Does it have measurable variables, and can it be shown to be false?
- What do people hope to learn from the experiments?
- Buy-in
- What specific changes would be made on the basis of the results?
- How will the organization ensure that the results aren’t ignored?
- How does the experiment fit into the organization’s overall learning agenda and strategic priorities?
- Feasibility
- Does the experiment have a testable prediction?
- What is the required sample size? Note: The sample size will depend on the expected effect (for example, a 5 percent increase in sales).
- Can the organization feasibly conduct the experiment at the test locations for the required duration?
- Reliability
- What measures will be used to account for systemic bias, whether it’s conscious or unconscious?
- Do the characteristics of the control group match those of the test group?
- Can the experiment be conducted in either “blind” or “double-blind” fashion?
- Have any remaining biases been eliminated through statistical analyses or other techniques?
- Would others conducting the same test obtain similar results?
- Causality
- Did we capture all variables that might influence our metrics?
- Can we link specific interventions to the observed effect?
- What is the strength of the evidence? Correlations are merely suggestive of causality.
- Are we comfortable taking action without evidence of causality?
- Value
- Has the organization considered a targeted rollout—that is, one that takes into account a proposed initiative’s effect on different customers, markets, and segments—to concentrate investments in areas when the potential payback is the highest?
- Has the organization implemented only the components of an initiative with the highest return on investment?
- Does the organization have a better understanding of what variables are causing what effects?
- Decisions
- Do we acknowledge that not every business decision can or should be resolved by experiments? But everything that can be tested should be tested.