When Experimentation and Alignment Collide
At Optimizely, our product team meets each week to discuss experiments we plan to run in our products.
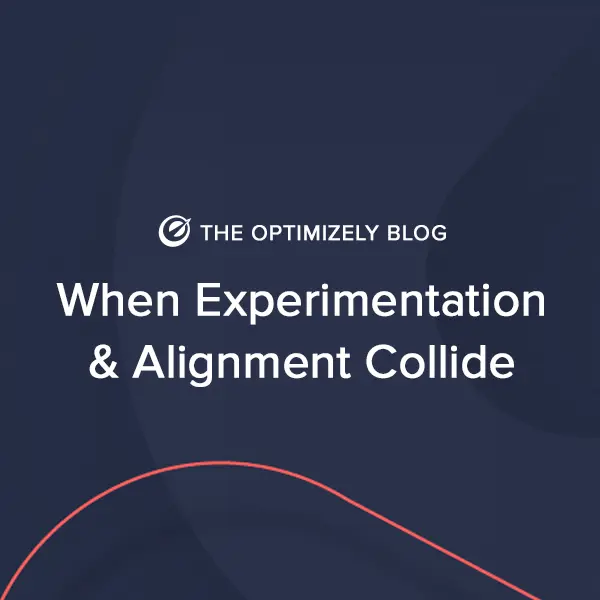
It’s an open meeting called “Experiment Review” and we use it to share best practices and ensure we’re running high quality experiments. This week in Experiment Review a debate sprung up around an experiment proposal. This debate revealed a cultural tension between:
- Encouraging individuals to validate their ideas with experiments
- Encouraging the organization to focus on a set of product priorities.
The debate started after two of our designers, Ron and Shane, proposed an experiment to the group. They had an idea for a novel way to increase a specific kind of user engagement with a new product we recently launched1.
On one side of the debate were several folks who pointed out that we didn’t have data to indicate that moving that particular metric would have a big impact on our business. They argued that this experiment was fixing a problem that we weren’t sure existed, thereby distracting us from fixing other problems that very much did.
On the other side were folks who argued that the team had made a good-faith effort to be experiment-driven and that by pushing back on this experiment we were discouraging that behavior. As our VP of Product, Claire, put it, we were weighing the cost of putting experimentation energy into a low-yield area against the cost of setting the experimentation bar so high that no one does it.
We spent some time discussing this, and here are some of the lessons we took away from this debate:
1. In an experimentation culture, leaders focus on prioritizing metrics
This debate made it clear we hadn’t done enough to communicate what our most important product problems are, and how our teammates could think about solving them. At Optimizely, we use high-level product metric targets (written as departmental OKRs) to accomplish this.
The challenge with high-level metric targets is that they aren’t always easy to tie back to an individual’s day-to-day work. They communicate our most important product problems, but they don’t always give our teammates an obvious way to think about solving them. One way to address this is to break your high-level metrics into a “goal tree” of sub-metrics. Here’s a simple example:
A shared breakdown of our top-level product OKRs into sub-problems would have given our teammates a framework for thinking about them without restricting the creativity of their ideas.
2. The level of rigor involved in qualifying a hypothesis should match the level of effort required to run the experiment
At Optimizely, one way we use experiments is as a low-effort way of validating a hypothesis before committing to building a feature. While it’s true that these experiments generally require less effort than the feature they’re intended to validate, they still take time and effort from multiple teammates. Interesting ideas are never in short supply here, so we run the risk of spending all of our time running experiments at the expense of shipping valuable products and features to our customers.
One way to work around this challenge is to set the expectation that the harder it is to run an experiment, the more time we should spend up front validating the hypothesis with customer data. Hypotheses should be backed up with analytics, customer interviews, and a compelling narrative the connects the hypothesis with the team’s priorities. The data, narrative, and proposed experiment design should be subject to peer review (such as Idea Scoring in Optimizely Program Management!).
This is the same type of work a good PM or PMM would use to prioritize a new project even if they weren’t planning on running an experiment. Indeed, validation experiments are just one step in the process of de-risking product development!
3. Setting the bar high encourages experimentation
There’s a pervasive myth in the tech industry that running experiments is akin to “throwing a bunch of stuff at the wall and seeing what sticks”. I’ve spent a lot of time talking with the teams who helped build the experimentation cultures at the world’s most sophisticated technology companies, and I believe this notion couldn’t be further from the truth. In these organizations, hypotheses and experiment design are subject to a thorough review process before they are tested “in the wild”.
Subjecting experiments to critical peer review before they are run might seem discouraging. It is, after all, another organizational hoop we’re asking our teammates to jump through. That may be true in the short term, but in the long term I suspect we’ll see exactly the opposite effect. Netflix and AirBnb, for example, each run thousands of experiments each year in spite of the fact that many experiment ideas face a gauntlet of organizational review before shipping.
Peer review forces experimenters to hone their hypotheses and experiments and helps ensure that the experiments we run have the best chance of making an impact on the business. Ultimately this will lead to more meaningful success stories in the organization. These successes encourage further experimentation.
So set the bar high and push your teammates to think hard about their experiments. Encourage your teammates to stretch their creativity and construct compelling, data-backed narratives for how their ideas will move the business forward, and make sure they have the necessary context to do that.
Following our discussion, Ron and Shane have gone to work validating their hypothesis. They’ve already managed to dig up a few customer quotes that support their thesis. I’m looking forward to seeing what they’ve come up with at Experiment Review next week!
1. For readers who are familiar with Optimizely Program Management: their hypothesis was that giving our customers a way to catalog “Product Problems” would encourage their Team members to create more Ideas.